Congratulations to Lixin Yuan on ICML 2025 (CCF-A) Paper Acceptance
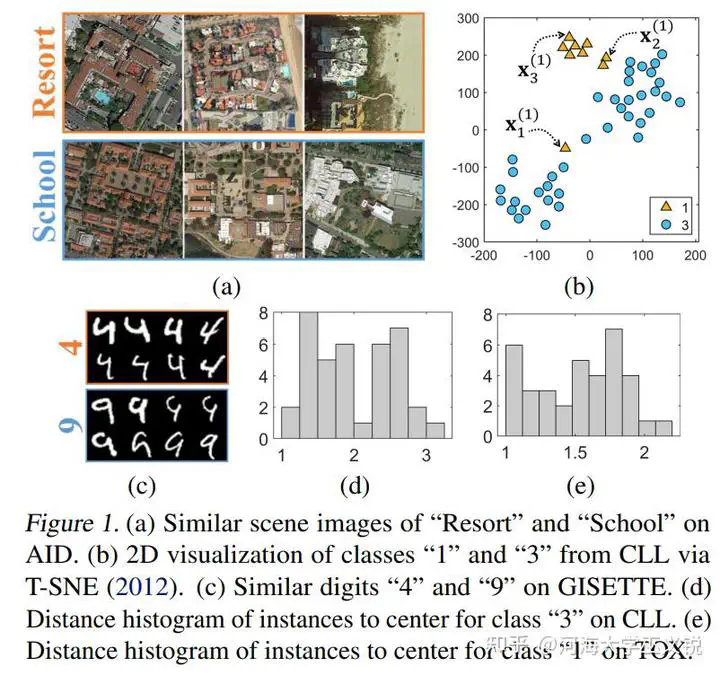
Stray Intrusive Outliers-Based Feature Selection on Intra-Class Asymmetric Instance Distribution or Multiple High-Density Clusters ICML2025 (CCF-A)
For data characterized by intra-class asymmetric instance distribution or multiple high-density clusters (ADMHC), traditional outliers hold genuine classification significance and encapsulate specific pattern features, yet their class main body often remains challenging to effectively identify. Existing feature selection (FS) methods predominantly perform feature scoring based on all training instances, rarely targeting intra-class ADMHC data specifically. To address this, this paper proposes a supervised feature selection method, SIOFS (Feature Selection based on Stray Invasive Outliers), which identifies the class main body by refining the skewness coefficient and integrating a 3σ criterion threshold, and subsequently evaluates feature importance based on the invasive degree of stray invasive outliers (SIOs). Additionally, an optimized density mean center is introduced to effectively represent the common characteristics of the class main body. Through mathematical modeling, theorem proving, and logical deduction, the theoretical rationality and universality of parameter settings in SIOFS are rigorously ensured. Experiments on 15 diverse benchmark datasets demonstrate that SIOFS significantly outperforms 12 state-of-the-art feature selection methods in classification accuracy, normalized mutual information (NMI), and confusion matrix metrics.